
1. ML Courses
1. Machine Learning Best
“Machine Learning” by DeepLearning.AI is a foundational online course taught by Andrew Ng on Coursera. It is one of the most popular and beginner-friendly machine learning courses.
Course Overview
- Focus: Covers the basics of machine learning with a practical, hands-on approach.
- Language: Uses Python and Octave/MATLAB for coding exercises.
- Prerequisites: Basic knowledge of linear algebra, probability, and programming is helpful but not mandatory.
Key Topics
- Supervised Learning (Linear regression, logistic regression, neural networks, support vector machines)
- Unsupervised Learning (K-means clustering, principal component analysis)
- Machine Learning Best Practices (Bias-variance tradeoff, error analysis, feature engineering)
Who Is It For?
- Beginners looking to enter the field of ML and AI
- Engineers, researchers, and professionals seeking practical ML skills
2. Stanford CS229: Machine Learning

Stanford CS229: Machine Learning is one of the most well-known machine learning courses, taught by Andrew Ng. It covers the theoretical foundations and practical applications of machine learning, focusing on mathematical rigor and real-world implementation.
Course Highlights
-
Core Topics:
- Supervised learning (linear regression, logistic regression, SVMs, neural networks)
- Unsupervised learning (PCA, k-means, Gaussian mixtures)
- Reinforcement learning and Markov decision processes
- Probabilistic graphical models
- Debugging ML Models
-
Prerequisites:
- Strong background in linear algebra, probability, and statistics
- Good programming skills (usually Python, MATLAB, or Octave)
-
Course Format:
- Lectures, assignments, and a final project where students apply ML techniques to real-world problems
3. Fast Ai Popular
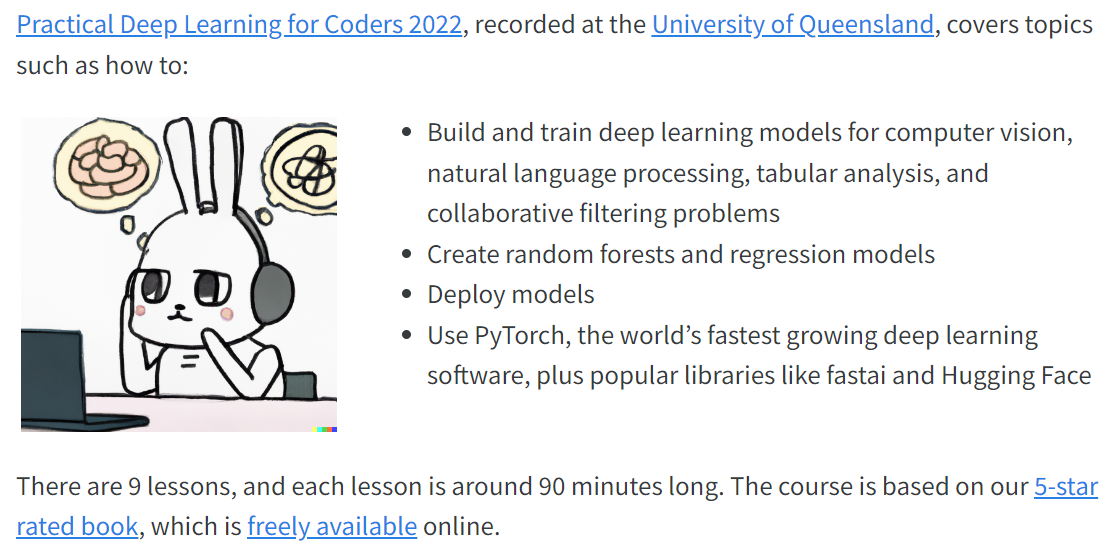
A free course designed for people with some coding experience, who want to learn how to apply deep learning and machine learning to practical problems.
Part 01
- 1: Getting started
- 2: Deployment
- 3: Neural net foundations
- 4: Natural Language (NLP)
- 5: From-scratch model
- 6: Random forests
- 7: Collaborative filtering
- 8: Convolutions (CNNs)
- …
Part 02
- 9: Stable Diffusion
- 10: Diving Deeper
- 11: Matrix multiplication
- 12: Mean shift clustering
- 13: Backpropagation & MLP
- 14: Backpropagation
- 15: Autoencoders
- 16: The Learner framework
- 17: Initialization/normalization
- 18: Accelerated SGD & ResNets
- 19: DDPM and Dropout
- 20: Mixed Precision
- …
3. Applied Machine Learning
To learn some of the most widely used techniques in ML:
- Optimization and Calculus
- Overfitting and Underfitting
- Regularization
- Monte Carlo Estimation
- Maximum Likelihood Learning
- Nearest Neighbours
- …

4. Machine Learning with Graphs (Stanford)
To learn some of the latest graph techniques in machine learning:
- PageRank
- Matrix Factorizing
- Node Embeddings
- Graph Neural Networks
- Knowledge Graphs
- Deep Generative Models for Graphs
- …

5. Probabilistic Machine Learning
To learn the probabilistic paradigm of ML:
- Reasoning about uncertainty
- Continuous Variables
- Sampling
- Markov Chain Monte Carlo
- Gaussian Distributions
- Graphical Models
- Tuning Inference Algorithms
- …

6. Deep Learning: CS 182
To learn some of the widely used techniques in deep learning:
- Machine Learning Basics
- Error Analysis
- Optimization
- Backpropagation
- Initialization
- Batch Normalization
- Style transfer
- Imitation Learning
- …

7. Deep Unsupervised Learning
To learn the latest and most widely used techniques in deep unsupervised learning:
- Autoregressive Models
- Flow Models
- Latent Variable Models
- Self-supervised learning
- Implicit Models
- Compression
- …
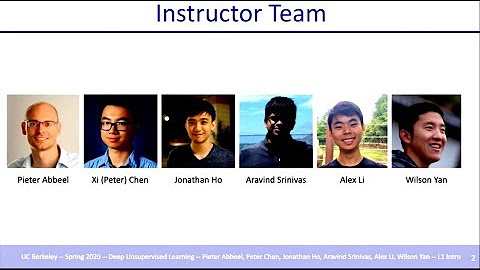
8. NYU Deep Learning SP21
To learn some of the advanced techniques in deep learning:
- Neural Nets: rotation and squashing
- Latent Variable Energy Based Models
- Unsupervised Learning
- Generative Adversarial Networks
- Autoencoders
- …
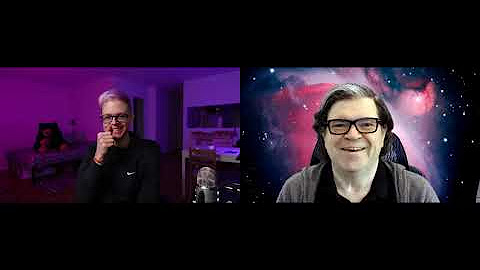
9. CMU Neural Networks for NLP
To learn the latest neural network based techniques for NLP:
- Language Modeling
- Efficiency tricks
- Conditioned Generation
- Structured Prediction
- Model Interpretation
- Advanced Search Algorithms
- …

10. Multilingual NLP
To learn the latest concepts for doing multilingual NLP:
- Typology
- Words, Part of Speech, and Morphology
- Advanced Text Classification
- Machine Translation
- Data Augmentation for MT
- Low Resource ASR
- Active Learning
- …
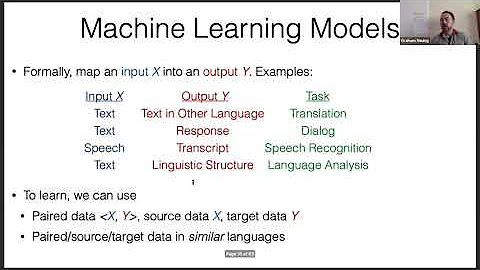
11. Advanced NLP
To learn advanced concepts in NLP:
- Attention Mechanisms
- Transformers
- BERT
- Question Answering
- Model Distillation
- Vision + Language
- Ethics in NLP
- Commonsense Reasoning
- …

12. Deep Learning for Computer Vision
To learn some of the fundamental concepts in CV:
- Introduction to deep learning for CV
- Image Classification
- Convolutional Networks
- Attention Networks
- Detection and Segmentation
- Generative Models
- …
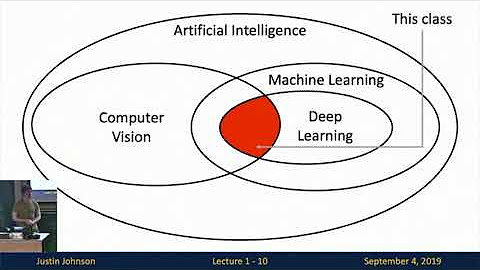
13. Deep Reinforcement Learning
To learn the latest concepts in deep RL:
- Intro to RL
- RL algorithms
- Real-world sequential decision making
- Supervised learning of behaviors
- Deep imitation learning
- Cost functions and reward functions
- …

14. Full Stack Deep Learning
To learn full-stack production deep learning concepts:
- ML Projects
- Infrastructure and Tooling
- Experiment Managing
- Troubleshooting DNNs
- Data Management
- Data Labeling
- Monitoring ML Models
- Web deployment
- …

Need Extra Help?